dropless moe|GitHub : Bacolod MegaBlocks is a light-weight library for mixture-of-experts (MoE) training. The core of the system is efficient "dropless-MoE" (dMoE, paper) and standard MoE layers. . Soban K-Town Grill 소반 - SM Megamall, Mandaluyong BBQ, Korean restaurant #12 of 4971 places to eat in Mandaluyong. Open until 10PM. . One of the best samgyeopsal so far. 100% cravings satisfied $$$$ Masil Korean Charcoal Grill Megamall Restaurant, BBQ #1159 of 4971 places to eat in Mandaluyong.
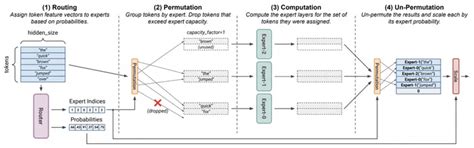
dropless moe,MegaBlocks is a light-weight library for mixture-of-experts (MoE) training. The core of the system is efficient "dropless-MoE" (dMoE, paper) and standard MoE layers. .MegaBlocks is a light-weight library for mixture-of-experts (MoE) training. The core of the system is efficient "dropless-MoE" ( dMoE , paper ) and standard MoE layers. .• We show how the computation in an MoE layer can be expressed as block-sparse operations to accommodate imbalanced assignment of tokens to experts. We use this .
MegaBlocks is a light-weight library for mixture-of-experts (MoE) training. The core of the system is efficient "dropless-MoE" ( dMoE , paper ) and standard MoE layers. .MegaBlocks is a light-weight library for mixture-of-experts (MoE) training. The core of the system is efficient "dropless-MoE" ( dMoE , paper ) and standard MoE layers. MegaBlocks is built on top of Megatron-LM , where we support data, .
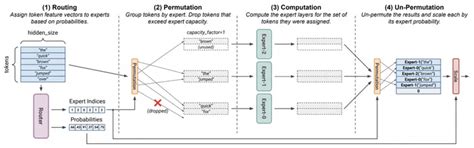
In contrast to competing algorithms, MegaBlocks dropless MoE allows us to scale up Transformer-based LLMs without the need for capacity factor or load balancing losses. .
GitHub Finally, also in 2022, “Dropless MoE” by Gale et al. reformulated sparse MoE as a block-sparse matrix multiplication, which allowed scaling up transformer models without the .The Mixture of Experts (MoE) models are an emerging class of sparsely activated deep learning models that have sublinear compute costs with respect to their parameters. In .
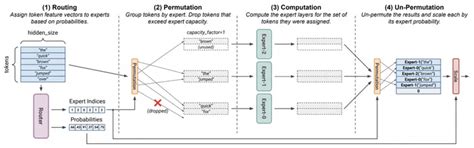
Abstract: Despite their remarkable achievement, gigantic transformers encounter significant drawbacks, including exorbitant computational and memory footprints during training, as .
dropless moe|GitHub
PH0 · megablocks · PyPI
PH1 · [2109.10465] Scalable and Efficient MoE Training for Multitask
PH2 · Towards Understanding Mixture of Experts in Deep Learning
PH3 · Sparse MoE as the New Dropout: Scaling Dense and Self
PH4 · MegaBlocks: Efficient Sparse Training with Mixture
PH5 · GitHub
PH6 · Efficient Mixtures of Experts with Block
PH7 · Aman's AI Journal • Primers • Mixture of Experts
PH8 · A self